For several years, organisations have been urged to become data driven. The news has recently moved on to talk about customer insights (CI) driven organisations. What has changed and what is the difference?
The conversation has changed because we have reached a tipping point in the maturity and productisation of several forms of artificial intelligence (AI), which is a game changer in the way we work and do business. Forrester has published a paper which explains why “2017 will be the year when businesses gain direct access to powerful customer insight via new cognitive interfaces and other AI-related tech. CI will drive change across the enterprise”. Forrester believes companies will promote business intelligence and data analytics to become a core strategy. Artificial Intelligence is being incorporated into Business Intelligence (BI) and analytics tools enabling business managers and users to apply them directly without the need to refer to data scientists and statisticians. They believe it will enable companies to transform themselves into true insights driven enterprises, a move that will leave any competitors that do not make the shift far behind.
The following graph is re-produced in the Forrester study from the Economic Intelligence Unit predicting a 27% annual growth rate for customer insights driven companies between 2015 and 2020, compared with any others which are expected to follow the typical growth rate in GDP of 3.5% per annum. The changes will see the 40 leading customer insights driven companies surveyed to increase revenue from US$333 billion in 2015 to US $1.2 trillion in 2020. The changes will see companies increase their investment in AI by 300% in 2017.
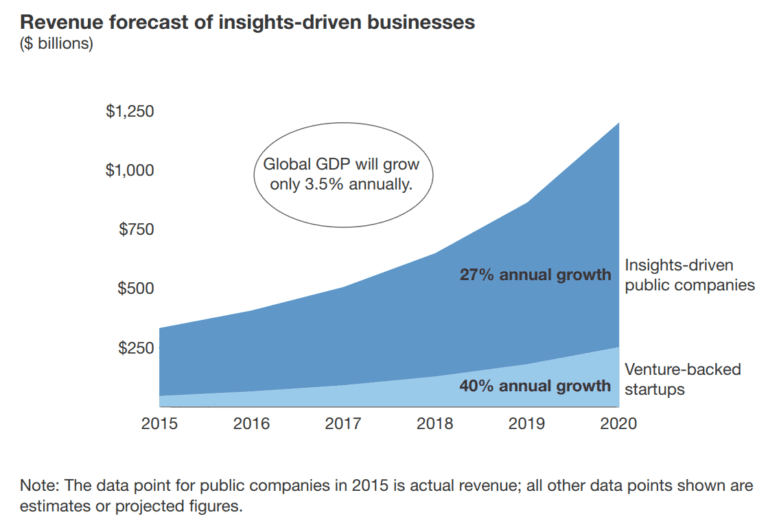
Insights-driven businesses will steal $1.2 trillion annually by 2020
What are the new trends?
A survey of 235 business executives in the USA by the National Business Research Institute (NBRI) in 2016 concluded that “38% of enterprises are already using AI technologies and 62% will use AI technologies by 2018,” according to Forbes.
To understand what is going on we need to look at several trends that are happening in parallel:
- Machine Learning is giving computers the ability to learn without being explicitly programmed. It is being incorporated into more applications, especially into the BI and analytics area, which enables pattern recognition to be executed independently of the user, so that the results provide advice directly to the user. Any enterprise applications involving prediction and classification are expected to use machine intelligence. Specialised forms can also use Deep Learning Platforms incorporating neural networks that interrogate data on multiple abstraction layers.
- Natural Language Processing is also being increasingly incorporated into BI and analytics tools. It can take the form of language generation (writing text), speech recognition or text analysis. Queries to software will continue to move towards natural language interfaces.
- Virtual Agents are implementations of these technologies, from simple chatbots to smart software that can interact with humans. Famous examples are Google Now, Siri and Cortana. However, often they are silent interactive software that can, for example, help customers complete application forms by pre completing information already known.
- The Internet of Things (IOT) is helping the trend with vendors embedding sensors in more connected hardware and software to enable data collection and tracking. It is creating new sources of data that can be analysed for patterns to understand customer activities in more detail.
- Cloud Services enable large quantities of enterprise data to be stored cheaply and made available for analysis. Deployment can be fast and cost effective with the company only paying for the services it uses. Third party data is also available to be merged with company big data.
- IBM’s Watson cognitive computing application is available as a service, including on the cloud, where it can be deployed to analyse enterprise data for patterns that return as advice to users. It is considered the original classic example of AI as a service. Many other vendors are emulating this platform with their own offerings designed to compete for the same corporate customers. Leading competitors are Google, Microsoft, Amazon, SAS and more, including many niche new start up players.
- Moore’s Law is marching on as always with computing power increasing, while falling in price. Ray Kurzweil has predicted that the same raw processing power that is estimated to be available in the human brain (20 quadrillion calculations per second) will be available by 2019 for US$4,000. This will enable AI applications to be feasible in mobile platforms such as pads and smartphones
What should companies be doing to become a Customer Insights driven organisation?
The changes will be so profound for a company that the decision to change to a customer insights driven organisation must come from the C-level managers. A strategy must first be formulated to exploit these trends within the unique context of the organisation. If it originates any lower a siloed parochial solution will emerge hidden within existing processes. The real benefits of radical change will be lost, except perhaps for an increase in performance of the local processes.
McKinsey in their 2015 paper An Executive’s Guide to Machine Learning, suggested there were three roles in the strategy: data scientists, business users and a new critical additional role of translators: “Translators can bridge the disciplines of data, machine learning, and decision making by reframing the…complex results as actionable insights that generalist managers can execute.” Other than this, the advice given for moving to a customer insights capability is similar to any BI or analytics data management strategy.
AI platforms such as IBM’s Watson and others ride on top of the company data assets, which they analyse to derive their conclusions and advice. The recommendations will therefore only be as good as the quality of the data they consume. The strategy depends upon the same critical success factors as any BI and analytics strategy. The organisation must be able to manage its own data effectively.
The diagram below from a McKinsey Global Institute report shows how different industries are applying AI to gain commercial advantage.
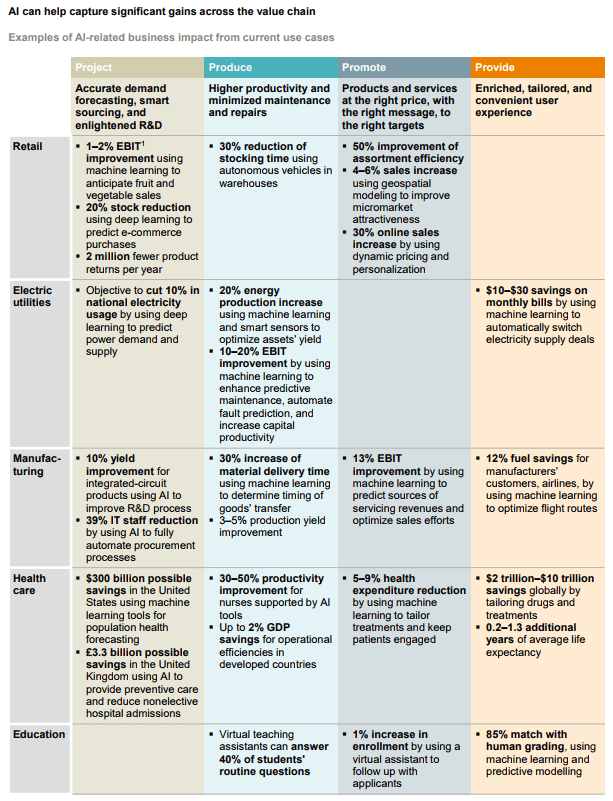
How should we approach the Strategy?
The Big No No
The organistaion strategy often begins by acquitting the technology first. Software vendors are only too willing to help with the process. The organisation then hire analytical skills and train their own personnel in analytics and how to use their new tools. This approach can be counter productive, committing the organisation to a solution without a complete understanding of the requirements.
Start here
Before diving into the technology, the organisation must be positioned to be able to identify and prepare the correct core data that must be used in the analytics process. If the organisation focuses upon the wrong data, they will not be able to answer their questions.
Quality Counts
Once the data is found the quality must be tested. If the data is of poor quality it may not be able to be analysed by the tools, or even worse if it can be analysed it may give the wrong answers. There will also be gaps in the data that must be filled. Data Assets should be pooled and shared, not hoarded by one department.
Apply the Basics
There remains a need for due diligence in managing the data. The data strategy, data governance, enterprise data model, data architecture, data dictionary and other standard resources are still required. Remember that data gathering, management and usage must still comply with governance, privacy and security standards. The best data must also be identified and sourced from operational systems for analysis, analytics and decision making. Organisations must be as diligent and successful at managing their data assets for AI as much as for BI. The same rules apply to both.
Change Culture
When an organisation has acquired the technology and analytical skills, and even obtained the results of their analysis, the new-found knowledge may not be acted upon. Business managers may not trust or act upon the analysis results unless they have been trained to depend upon data in their decision making. The organisation needs to undergo a corporate cultural change into a data centric organisation that intuitively relies upon data in their decision-making processes. Business managers once armed with valuable insights must be encouraged to follow through implementing changes based upon them.
Start Small
As with any strategy we must start small, aim for low hanging fruit first and grow as successes are achieved. AI must be applied in easy stages. The first step is to use it to describe what is happening. Once experience is gained, a prediction of what will happen and what should be done to exploit it can be obtained.
Getting Smarter
Prediction and prescription are the stages of the strategy that are most sensitive to data quality, and this can be a major concern to managers who do not want to proceed further. Experience has proven that as companies gain experience they also develop confidence in drawing conclusions from messy data assets, although this is no excuse for not doing everything possible to improve that quality.
The graph below from a Raconteur report shows how mature different industries are in their AI investments.
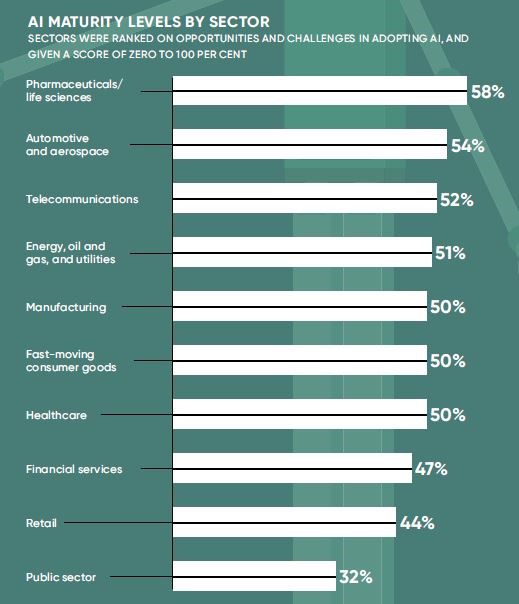
What does a customer insight driven organisation look like?
Finally, we need to ask this question so we have some idea where we are headed, have some idea when we are on target and when we have arrived. We cannot see what the perfect, completely customer insights and data driven organisation looks like yet. Some of the best examples are the companies we have already mentioned as AI vendors, who also depend upon information for their existence, such as Google, Amazon and Microsoft. There are seven characteristics we can look for in such organisations:
- They will have a well implemented data strategy that records and acquires data from all interactions with the customer on all channels.
- Useful third-party data will be regularly acquired and routinely merged with corporate data to augment the base assets which are analysed.
- Business users at all levels of the organisation will expect data to be available either in ready to use form, or easily sourced for all decision-making processes.
- The data will be available in real time or with very short latency.
- It will be inherent within the corporate culture to base all decisions and support all processes with data analytics.
- Business users will work in collaboration with AI analysis and recommendations.
- Business processes will be based upon Agile methodologies.
The quadrants below are based upon a model from Accenture shows different types of applications that can emerge from AI investments. They vary from simple automation in the bottom left to creative augmentation in the top right of the quadrant in a comparison of data and work complexity.
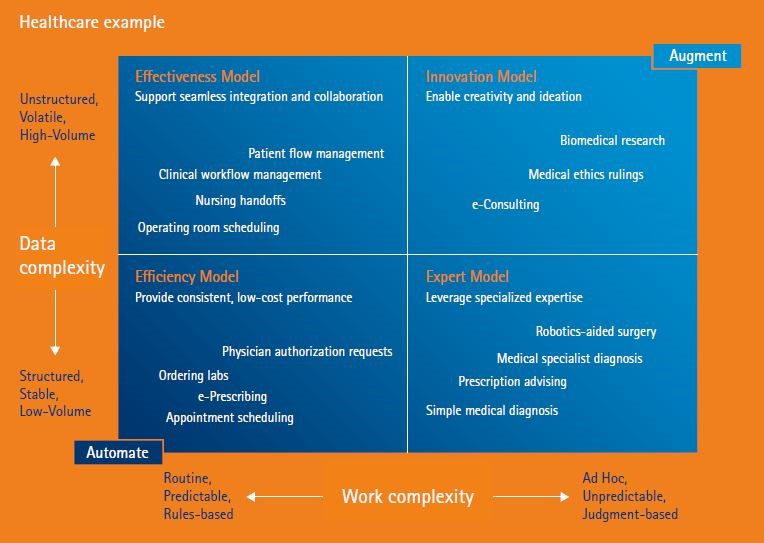
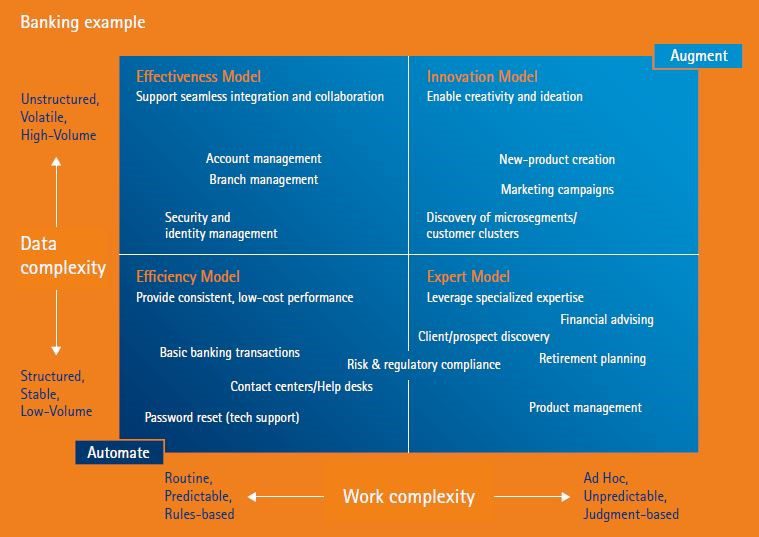
What does this future look like?
In the future, we will find out where all of this is heading. Speculation points to the decision-making process progressively becoming more dependent upon AI applications once we gain experience and confidence. The speed of analysis and decision making may increase and be too fast for humans to be part of the operational process. Human involvement may be withdrawn from the process altogether. Then companies will set up what McKinsey have called Distributed Autonomous Corporations. The daily operational decisions will be automated and managers would audit the results and periodically re-configure the software to change the strategic direction when required.
Conclusion
Organisations that want to succeed in the medium term need to be embracing the opportunities technology is offering now. Some organisations are off and running already. McKinsey believe that catching up will not be possible, but all evidence is that some companies are catching up once they are truly committed. The organisation must have a CI strategy supported from the most senior level to succeed because the whole organisation will be changed and impacted. In the past companies have bought the technology and passively expected change. This has not worked with less fundamental changes in the past and will not succeed with these more profound changes. Companies that are not customer insights driven will be left behind as they lose customers.
Accenture, Turning Artificial Intelligence into Business Value
https://www.accenture.com/t20160814T215045Z__w__/us-en/_acnmedia/Accenture/Conversion-Assets/DotCom/Documents/Global/PDF/Technology_11/Accenture-Turning-Artificial-Intelligence-into-Business-Value.pdf
Forbes, 2017, “Top 10 Hot Artificial Intelligence (AI) Technologies
https://www.forbes.com/sites/gilpress/2017/01/23/top-10-hot-artificial-intelligence-ai-technologies/#224f51801928
Forbes, 2017, “Artificial Intelligence Rapidly Adopted By Enterprises, Survey Says”
https://www.forbes.com/sites/gilpress/2016/07/20/artificial-intelligence-rapidly-adopted-by-enterprises-survey-says/#6a23481212da
Forrester, 2017, “Forrester Predictions 2017 Artificial Intelligence Will Drive The Insights Revolution”
https://go.forrester.com/wp-content/uploads/Forrester_Predictions_2017_-Artificial_Intelligence_Will_Drive_The_Insights_Revolution.pdf
McKinsey, 2015, “An Executive’s Guide to Machine Learning”
http://www.mckinsey.com/industries/high-tech/our-insights/an-executives-guide-to-machine-learning
McKinsey Global Institute, 2017, Artificial Intelligence The Next Digital Frontier
https://www.google.com.au/searchq=McKinsey+Global+Institute%2C+2017%2C+Artificial+Intelligence+The+Next+Digital+Frontier&oq=McKinsey+Global+Institute%2C+2017%2C+Artificial+Intelligence+The+Next+Digital+Frontier&aqs=chrome..69i57.2097j0j8&sourceid=chrome&ie=UTF-8Raconteur, 2017,
Artificial Intelligence for Business
https://www.raconteur.net/artificial-intelligence-for-business
Ray Kurzweil, 2016 “Predictions by Ray Kurzweil” on Moore’s Law
https://en.wikipedia.org/wiki/Predictions_made_by_Ray_Kurzweil